Options
Variance-based Clustering Technique for Distributed Data Mining Applications
Author(s)
Date Issued
2007-06-28
Date Available
2016-09-02T10:12:20Z
Abstract
Nowadays, huge amounts of data are naturally collected in distributed sites due to different facts and moving these data through the network for extracting useful knowledge is almost unfeasible for either technical reasons or policies. Furthermore, classical parallel algorithms cannot be applied, specially in loosely coupled environments. This requires to develop scalable distributed algorithms able to return the global knowledge by aggregating local results in an effective way. In this paper we propose a distributed algorithm based on independent local clustering processes and a global merging based on minimum variance increases and requires a limited communication overhead. We also introduce the notion of distributed sub-clusters perturbation to improve the global generated distribution. We show that this algorithm improves the quality of clustering compared to classical local centralized ones and is able to find real global data nature or distribution.
Type of Material
Conference Publication
Publisher
CSREA Press
Web versions
Language
English
Status of Item
Peer reviewed
Journal
Proceedings of the 2007 International Conference on Data Mining, DMIN 2007, June 25-28, 2007, Las Vegas, Nevada, USA
Conference Details
2007 International Conference on Data Mining (DMIN'07), Las Vegas, Nevada, USA, 25-28 June 2007
ISBN
978-1-60132-031-0
This item is made available under a Creative Commons License
File(s)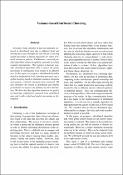
Loading...
Name
paperAIPR07.pdf
Size
250.4 KB
Format
Adobe PDF
Checksum (MD5)
471a2cdf392768623eef8f2cc78f0818
Owning collection