Options
Quantifying the scalability of reduced-order grey-box energy models for commercial building stock modeling
Date Issued
2021-06-16
Date Available
2024-06-06T11:48:15Z
Abstract
Grey-box models are extensively employed in building energy simulations. However, the grey-box approach often leads to application and stakeholder specific models, for instance, the design approach of greybox modeling for commercial buildings differs on a case by case basis. Often, the network order limits the scalability of these networks. Reduced-order grey-box modeling approaches counter these limitations by achieving a trade off between model complexity and desired accuracy. This study, therefore, formulates a generalized methodology to quantify scalability associated with reduced-order grey-box models for heat demand modeling of commercial buildings. The devised methodology assesses model scalability through (1) scalability feature-definition, (2) model identification, (3) multi-level modeling and (4) KPI identification procedures. This study formulates a test-case of 10 buildings (on university campus) with varied operations to implement the devised methodology. Results indicate that model scalability directly associates with the nature of building operation. Furthermore, similar zone variables can effectively represent an entire building provided that the considered zone pre-dominantly occupies majority of the building’s indoor space.
Sponsorship
University College Dublin
Science Foundation Ireland
Type of Material
Conference Publication
Publisher
IBPSA Canada
Web versions
Language
English
Status of Item
Peer reviewed
Journal
Proceedings of eSim 2020: 11th Conference of IBPSA-Canada
Conference Details
The 11th Conference of International Building Performance Simulation Association-Canada (IBPSA-Canada) (eSim2020), Vancouver, Canada, 14-16 June 2021
This item is made available under a Creative Commons License
File(s)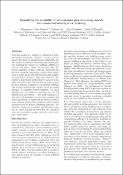
Loading...
Name
esim2020_1132.pdf
Size
535.65 KB
Format
Adobe PDF
Checksum (MD5)
93713a879e7a7eeb3ad091d4fef428b0
Owning collection
Mapped collections