Options
Bayesian variable selection for latent class analysis using a collapsed Gibbs sampler
Author(s)
Date Issued
2016-01
Date Available
2019-07-10T10:36:05Z
Abstract
Latent class analysis is used to perform model based clustering for multivariate categorical responses. Selection of the variables most relevant for clustering is an important task which can affect the quality of clustering considerably. This work considers a Bayesian approach for selecting the number of clusters and the best clustering variables. The main idea is to reformulate the problem of group and variable selection as a probabilistically driven search over a large discrete space using Markov chain Monte Carlo (MCMC) methods. Both selection tasks are carried out simultaneously using an MCMC approach based on a collapsed Gibbs sampling method, whereby several model parameters are integrated from the model, substantially improving computational performance. Post-hoc procedures for parameter and uncertainty estimation are outlined. The approach is tested on simulated and real data.
Sponsorship
Science Foundation Ireland
Other Sponsorship
Insight Research Centre
Type of Material
Journal Article
Publisher
Springer
Journal
Statistics and Computing
Volume
26
Issue
1-2
Start Page
511
End Page
527
Copyright (Published Version)
2014 Springer
Language
English
Status of Item
Peer reviewed
This item is made available under a Creative Commons License
File(s)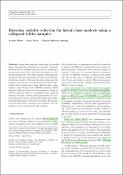
Loading...
Name
Bayesian variable selection for latent class analysis using a collapsed gibbs sampler.pdf
Size
702.24 KB
Format
Adobe PDF
Checksum (MD5)
ef3574d2b9b1e2e51977160d893feebe
Owning collection