Options
Exact and Hybrid Solutions for the Multi-objective VM Reassignment Problem
Date Issued
2017-02-23
Date Available
2018-02-01T02:00:14Z
Abstract
Machine Reassignment is a challenging problem for constraint programming (CP) and mixed integer linear programming (MILP) approaches, especially given the size of data centres. Hybrid solutions mixing CP and heuristic algorithms, such as, large neighbourhood search (CBLNS), also struggle to address the problem given its size and number of constraints. The multi-objective version of the Machine Reassignment Problem is even more challenging and it seems unlikely for CP, MILP or hybrid solutions to obtain good results in this context. As a result, the first approaches to address this problem have been based on other optimisation methods, including metaheuristics. In this paper we study three things: (i) under which conditions a mixed integer optimisation solver, such as IBM ILOG CPLEX, can be used for the Multi-objective Machine Reassignment Problem; (ii) how much of the search space can a well-known hybrid method such as CBLNS explore; and (iii) can we find a better hybrid approach combining MILP or CBLNS and another recent metaheuristic proposed for the problem (GeNePi). We show that MILP can handle only small or medium scale data centres, and with some relaxations, such as, an optimality tolerance gap and a limited number of directions explored in the search space. CBLNS on the other hand struggles with the problem in general but achieves reasonable performance for large instances of the problem. However, we show that our hybridisation improves both the quality of the set of solutions (CPLEX+GeNePi and CBLNS+GeNePi improve the solutions by +17.8% against CPLEX alone and +615% against CBLNS alone) and number of solutions (8.9 times more solutions than CPLEX alone and 56.76 times more solutions than CBLNS alone), while the processing time of CPLEX+GeNePi and CBLNS+GeNePi increases only by 6% and 16.4% respectively. Overall, the study shows that CPLEX+GeNePi is the best algorithm for small instances (CBLNS+GeNePi only gets 45.2% of CPLEX+GeNePi’s hypervolume) while CBLNS+GeNePi is better than the others on large instances (that CPLEX+GeNePi cannot address).
Sponsorship
Science Foundation Ireland
Other Sponsorship
Lero
Type of Material
Journal Article
Publisher
World Scientific Publishing
Journal
International Journal on Artificial Intelligence Tools
Volume
26
Issue
1
Copyright (Published Version)
2017 World Scientific Publishing Company
Language
English
Status of Item
Peer reviewed
This item is made available under a Creative Commons License
File(s)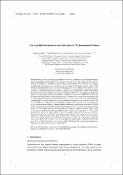
Loading...
Name
CPLEX_for_MOMRP_IJTAI_v1_BlackWhite_v2.pdf
Size
1.46 MB
Format
Adobe PDF
Checksum (MD5)
83fb5ae9e5d73b9c51fb74a1694df80c
Owning collection
Mapped collections