Options
Twin-Systems to Explain Artificial Neural Networks using Case-Based Reasoning: Comparative Tests of Feature-Weighting Methods in ANN-CBR Twins for XAI
Author(s)
Date Issued
2019-07-31
Date Available
2019-08-29T11:38:34Z
Abstract
In this paper, twin-systems are described to address the eXplainable artificial intelligence (XAI) problem, where a black box model is mapped to a white box “twin” that is more interpretable, with both systems using the same dataset. The framework is instantiated by twinning an artificial neural network (ANN; black box) with a case-based reasoning system (CBR; white box), and mapping the feature weights from the former to the latter to find cases that explain the ANN’s outputs. Using a novel evaluation method, the effectiveness of this twin-system approach is demonstrated by showing that nearest neighbor cases can be found to match the ANN predictions for benchmark datasets. Several feature-weighting methods are competitively tested in two experiments, including our novel, contributions-based method (called COLE) that is found to perform best. The tests consider the ”twinning” of traditional multilayer perceptron (MLP) networks and convolutional neural networks (CNN) with CBR systems. For the CNNs trained on image data, qualitative evidence shows that cases provide plausible explanations for the CNN’s classifications.
Sponsorship
Science Foundation Ireland
Other Sponsorship
Insight Research Centre
Type of Material
Conference Publication
Start Page
2708
End Page
2715
Copyright (Published Version)
2019 International Joint Conferences on Artificial Intelligence
Web versions
Language
English
Status of Item
Peer reviewed
Journal
Kraus, S. (ed.). Proceedings of the Twenty-Eighth International Joint Conference on Artificial Intelligence
Conference Details
Twenty-Eighth International Joint Conferences on Artifical Intelligence (IJCAI), Macao, 10-16 August 2019
This item is made available under a Creative Commons License
File(s)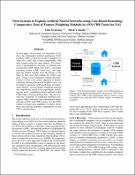
Loading...
Name
insight_publication.pdf
Size
680.59 KB
Format
Adobe PDF
Checksum (MD5)
59a5ce228d322c1efe17c974658e825c
Owning collection
Mapped collections