Options
Modeling sea-level change using errors-in-variables integrated Gaussian processes
Date Issued
2015-06
Date Available
2016-10-07T14:36:41Z
Abstract
We perform Bayesian inference on historical and late Holocene (last 2000 years) rates of sea-level change. The input data to our model are tidegauge measurements and proxy reconstructions from cores of coastal sediment. These data are complicated by multiple sources of uncertainty, some of which arise as part of the data collection exercise. Notably, the proxy reconstructions include temporal uncertainty from dating of the sediment core using techniques such as radiocarbon. The model we propose places a Gaussian process prior on the rate of sea-level change, which is then integrated and set in an errors-in-variables framework to take account of age uncertainty. The resulting model captures the continuous and dynamic evolution of sea-level change with full consideration of all sources of uncertainty. We demonstrate the performance of our model using two real (and previously published) example data sets. The global tide-gauge data set indicates that sea-level rise increased from a rate with a posterior mean of 1.13 mm/yr in 1880 AD (0.89 to 1.28 mm/yr 95% credible interval for the posterior mean) to a posterior mean rate of 1.92 mm/yr in 2009 AD (1.84 to 2.03 mm/yr 95% credible interval for the posterior mean). The proxy reconstruction from North Carolina (USA) after correction for land-level change shows the 2000 AD rate of rise to have a posterior mean of 2.44 mm/yr (1.91 to 3.01 mm/yr 95% credible interval). This is unprecedented in at least the last 2000 years.
Type of Material
Journal Article
Publisher
Institute of Mathematical Statistics
Journal
Annals of Applied Statistics
Volume
9
Issue
2
Start Page
547
End Page
571
Language
English
Status of Item
Peer reviewed
This item is made available under a Creative Commons License
File(s)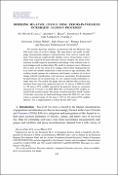
Loading...
Name
Cahill_SeaLevelAOAS_2015.pdf
Size
1.42 MB
Format
Adobe PDF
Checksum (MD5)
59c13f54b3277f2454e0fb1bce1d0b39
Owning collection
Mapped collections