Options
A Distributed Asynchronous Deep Reinforcement Learning Framework for Recommender Systems
Date Issued
2020-09-26
Date Available
2021-05-27T11:57:43Z
Abstract
In this paper we propose DADRL, a distributed, asynchronous reinforcement learning recommender system based on the asynchronous advantage actor-critic model (A3C), which combines ideas from A3C and federated learning (FL). The proposed algorithm keeps the user preferences or interactions on local devices and uses a combination of on-device, local recommendation models and a complementary global model. The global model is trained only by the loss gradients of the local models, rather than directly using user preferences or interactions data. We demonstrate, using well-known datasets and benchmark algorithms, how this approach can deliver performance that is comparable with the current state-of-the-art while enhancing user privacy.
Sponsorship
Science Foundation Ireland
Other Sponsorship
Insight Research Centre
Type of Material
Conference Publication
Publisher
ACM
Copyright (Published Version)
2020 Association for Computing Machinery
Web versions
Language
English
Status of Item
Peer reviewed
Journal
RecSys '20: Fourteenth ACM Conference on Recommender Systems
Conference Details
The 14th ACM Conference on Recommender Systems (RecSys 2020), Online, 22-26 September 2020
This item is made available under a Creative Commons License
File(s)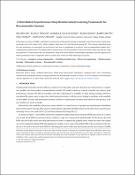
Loading...
Name
A Distributed Asynchronous Deep Reinforcement Learning Framework for Recommender Systems.pdf
Size
471.81 KB
Format
Adobe PDF
Checksum (MD5)
fa44e56b7d7c52ed21b61661f0081b01
Owning collection