Options
Protein Backbone Angle Prediction in Multidimensional φ-ψ Space
Date Issued
2006-01-20
Date Available
2021-07-28T15:20:01Z
Abstract
A significant step towards establishing the structure and function of a protein is the prediction of the local conformation of the polypeptide chain. In this article we present systems for the prediction of 3 new alphabets of local structural motifs. The motifs are built by applying multidimensional scaling (MDS) and clustering to pair-wise angular distances for multiple φ-ψ angle values collected from high-resolution protein structures. The predictive systems, based on ensembles of bidirectional recurrent neural network architectures, and trained on a large non-redundant set of protein structures, achieve 72%, 66% and 60% correct structural motif prediction on an independent test set for di-peptides (6 classes), tripeptides (8 classes) and tetra-peptides (14 classes), respectively, 28-30% above base-line statistical predictors. To demonstrate that structural motif predictions contain relevant structural information, we build a further system, based on ensembles of two-layered bidirectional recurrent neural networks, to map structural motif predictions into traditional 3-class (helix, strand, coil) secondary structure. This system achieves 79.5% correct prediction using the “hard” CASP 3-class assignment, and 81.4% with a more lenient assignment, outperforming a sophisticated state-of-the-art predictor (Porter) trained in the same experimental conditions. All the predictive systems will be provided free of charge to academic users and made publicly available at the address http://distill.ucd.ie/.
Sponsorship
Health Research Board
Irish Research Council for Science, Engineering and Technology
Science Foundation Ireland
Other Sponsorship
UCD President’s Award 2004
Type of Material
Technical Report
Publisher
University College Dublin. School of Computer Science and Informatics
Series
UCD CSI Technical Reports
ucd-csi-2006-1
Copyright (Published Version)
2006 the Authors
Language
English
Status of Item
Not peer reviewed
This item is made available under a Creative Commons License
File(s)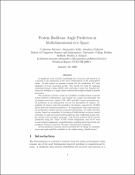
Loading...
Name
ucd-csi-2006-1.pdf
Size
243.78 KB
Format
Adobe PDF
Checksum (MD5)
707e890032e030a4ac78c0ef4d1bc511
Owning collection
Mapped collections