Options
A Bayesian deep-learning framework for assessing the energy flexibility of residential buildings with multicomponent energy systems
Date Issued
2023-10-15
Date Available
2024-06-06T14:42:49Z
Abstract
This paper addresses the challenge of assessing uncertainty in energy flexibility predictions, which is a significant open question in the energy flexibility assessment field. To address this challenge, a methodology that quantifies the flexibility of multiple thermal and electrical systems is developed using appropriate indicators and considers the different types of uncertainty associated with building energy use. A Bayesian convolutional neural network is developed to capture aleatoric and epistemic uncertainty related to energy conversion device operation and temperature deviations resulting from exploiting building flexibility. The developed prediction models utilise residential occupancy patterns and a sliding window technique and are periodically updated. The energy systems evaluated include a heat pump, a photovoltaic system, and a stationary battery, and use synthetic datasets obtained from a calibrated physics-based model of an all-electric residential building for two occupancy profiles. Simulation results indicate that building flexibility potential predictability is influenced by weather conditions and/or occupant behaviour. Furthermore, the day-ahead and hour-ahead prediction models show excellent performance for both occupancy profiles, achieving coefficients of determination between 0.93 and 0.99. This methodology can enable electricity aggregators to evaluate building portfolios, considering uncertainty and multi-step predictions, to shift electricity demand to off-peak periods or periods of excess onsite renewable electricity generation in an end-user-customised manner.
Sponsorship
Science Foundation Ireland
Other Sponsorship
UCD Energy Institute
Type of Material
Journal Article
Publisher
Elsevier
Journal
Applied Energy
Volume
348
Start Page
1
End Page
25
Copyright (Published Version)
2023 Elsevier
Language
English
Status of Item
Peer reviewed
ISSN
0306-2619
This item is made available under a Creative Commons License
File(s)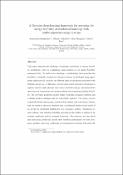
Loading...
Name
AB_ocr.pdf
Size
23.97 MB
Format
Adobe PDF
Checksum (MD5)
5978691dfc51f0b5fcef6dc46c7ee073-5
Owning collection
Mapped collections