Options
Latent space models for multiview network data
Author(s)
Date Issued
2017-09
Date Available
2018-04-17T12:23:06Z
Abstract
Social relationships consist of interactions along multiple dimensions. In social networks, this means that individuals form multiple types of relationships with the same person (an individual will not trust all of his/her acquaintances, for example). Statistical models for these data require understanding two related types of dependence structure: (i) structure within each relationship type, or network view, and (ii) the association between views. In this paper we propose a statistical framework that parsimoniously represents dependence between relationship types while also maintaining enough flexibility to allow individuals to serve different roles in different relationship types. Our approach builds on work on latent space models for networks (see Hoff et al. (2002), for example). These models represent the propensity for two individuals to form edges as conditionally independent given the distance between the individuals in an unobserved social space. Our work departs from previous work in this area by representing dependence structure between network views through a Multivariate Bernoulli likelihood, providing a representation of between-view association. This approach infers correlations between views not explained by the latent space model. Using our method, we explore 6 multiview network structures across 75 villages in rural southern Karnataka, India (Banerjee et al., 2013).
Type of Material
Journal Article
Publisher
Institute of Mathematical Studies
Journal
Annals of Applied Statistics
Volume
11
Issue
3
Start Page
1217
End Page
1244
Language
English
Status of Item
Peer reviewed
ISSN
1941-7330
This item is made available under a Creative Commons License
File(s)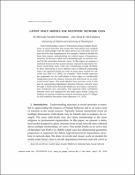
Loading...
Name
AOAS1411-022R2A0.pdf
Size
634.08 KB
Format
Adobe PDF
Checksum (MD5)
8dad0fc316df8b31f98fb372ad780b20
Owning collection