Options
Evaluating the effects of occupancy on energy use and indoor environmental quality in residential building archetypes over spatial and temporal scales
Author(s)
Date Issued
2025
Date Available
2025-02-19T13:08:48Z
Abstract
The European Union’s ambitious 2030 target to reduce energy consumption by 30% underscores the need for effective energy policies in the residential building sector. Accurate energy consumption estimation at various spatial and temporal scales is complex and computationally intensive. Archetypes simplify this task but often overlook critical factors such as occupancy uncertainty and the relationship between energy use and Indoor Environmental Quality (IEQ). This can lead to significant performance gaps between estimated and measured energy consumption. Addressing these challenges is essential for developing reliable decision-support tools and aligning energy estimates with measured energy usage. This thesis explores the impact of occupancy and the energy-IEQ relationship on energy consumption across multiple spatial and temporal scales, using Irish residential building archetypes as a case study. To achieve this, the thesis develops methodologies for creating various occupancy models, which are then integrated into residential archetypes to estimate energy consumption at different spatial and temporal scales. Furthermore, the correlation between energy consumption and IEQ is analysed to establish a balance between energy efficiency and maintaining healthy indoor environments. By deepening the understanding of occupancy and the energy-IEQ relationship, this study focuses on enhancing the capabilities and robustness of residential building archetypes for estimating energy consumption at multiple spatial and temporal scales through three distinct contributions to knowledge. The first contribution (Chapter 2) of this thesis focuses on developing a deterministic occupancy model, as a representative of a large number of dwellings, using Time Use Survey (TUS) data to generate day-wise occupancy schedules. This model is based on a specific set of rules, including the number of occupants, dwelling type, month of the year, and day of the week. The model converts the recorded activity in the TUS data into presence/absence profiles, providing insights into energy use related to daily activities. When validated against measured gas consumption data, the deterministic model demonstrates significant improvements in predicting heating energy consumption, with annual variations of approximately 10% and daily variations of up to 50% compared to standard occupancy models. Monthly heating and electricity consumption show variations of approximately 25% and 32%, respectively, compared to the base case. On a more granular level, daily variations in heating and electricity consumption are reported as 50% and 12%, respectively, compared to the base case with standard and conventional schedules. The second contribution (Chapter 3) devises a methodology to develop a Probabilistic Occupancy Model (UPOM), representative of a large number of dwellings, that is suitable for residential energy analysis over multiple spatial scales. The occupancy model is developed using Bayesian Neural Networks (BNNs) based on Time Use Survey (TUS) data. The multi-class classification model predicts four occupancy states, providing probability distributions that capture the uncertainty inherent in each prediction. The UPOM achieved 90% accuracy in predicting nighttime occupancy states and an average accuracy of 64% during the day, reflecting variations in daytime activities. Comparative analyses are conducted between the UPOM, a deterministic model, and standard occupancy models in terms of estimating heating energy consumption at various spatial and temporal scales. Statistical analyses using Analysis Of Variance (ANOVA) and Tukey’s honestly significant difference (Tukey HSD) tests confirmed the statistical significance of the differences among models, establishing UPOM as the most reliable for estimating energy consumption followed by the deterministic model. To further extend the capabilities of occupancy-based archetypes, the third contribution (Chapter 4) proposes a low-computational methodology based on a metamodel approach tailored for rapid prediction and optimisation of heating energy consumption (kWh), thermal discomfort (hours), and elevated CO2 levels (hours) under the influence of occupancy. The framework evaluates the impact of occupancy on the Pareto optimal front, balancing these three objectives for energy-efficient, naturally ventilated residential buildings in a temperate oceanic climate. For this purpose, a synthetic dataset is generated via parametric simulation using a validated EnergyPlus model of a representative dwelling archetype. The parametric simulation includes a range of occupancy-related parameters such as metabolic rates, occupancy density, clothing value and window operations, along with other building input parameters. The metamodel-based optimisation significantly reduces computation time by 80% compared to physicsbased optimisation, maintaining a high correlation coefficient of 0.98 between the two approaches. By including occupancy-related variables in the study, this study ensures that the predicted results and optimised design and operational parameters are resilient, and within acceptable limits, as recommended by the WHO and CIBSE TM59, whilst minimising the heating consumption to align with appropriate standards for energy-efficient homes. By integrating realistic occupancy behaviour and the strong relationship between energy consumption and IEQ, the study provides robust archetypes that enhance the prediction accuracy of energy consumption at multiple spatial and temporal scales. Policymakers can leverage these findings to refine and update building performance standards, ensuring they reflect real-world conditions and promote better compliance and implementation across the residential building sector. The enhanced predictive accuracy and reduced optimisation time facilitated by this research encourage the adoption of more efficient building management systems and retrofitting practices. This supports the development of sustainable building policies that prioritise both energy efficiency and IEQ in residential dwellings.
Type of Material
Doctoral Thesis
Publisher
University College Dublin. School of Mechanical and Materials Engineering and UCD Energy Institute
Qualification Name
Ph.D.
Copyright (Published Version)
2025 the Author
Language
English
Status of Item
Peer reviewed
This item is made available under a Creative Commons License
File(s)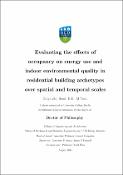
Loading...
Name
DivyanshuSood_19209633 2.pdf
Size
25.05 MB
Format
Adobe PDF
Checksum (MD5)
ca9e9731a892c46227308ac2be6f4e35
Owning collection