Options
Robust Model-Based Learning to Discover New Wheat Varieties and Discriminate Adulterated Kernels in X-Ray Images
Date Issued
2019-09-19
Date Available
2024-05-08T15:34:53Z
Abstract
In semi-supervised classification, class-memberships are learnt from a trustworthy set of units. Despite careful data collection, some labels in the learning set could be unreliable (label noise). Further, a proportion of observations might depart from the main structure of the data (outliers) and new groups may appear in the test set, that were not encountered earlier in the training phase (unobserved classes). Therefore, we present here a robust and adaptive version of the Discriminant Analysis rule, capable of handling situations in which one or more of the afore-mentioned problems occur. The proposed approach is successfully employed in performing anomaly and novelty detection on geometric features recorded from X-ray photograms of grain kernels from different varieties.
Sponsorship
Science Foundation Ireland
Other Sponsorship
Insight Research Centre
Science Foundation Ireland
Type of Material
Conference Publication
Publisher
Springer
Series
Studies in Classification, Data Analysis, and Knowledge Organization
Copyright (Published Version)
2021 Springer
Web versions
Language
English
Status of Item
Peer reviewed
Journal
Balzano, S. Porzio, G.C., Salvatore, R. et al. (eds.). Statistical Learning and Modeling in Data Analysis: Methods and Applications
Conference Details
The 12th Scientific Meeting of the Classification and Data Analysis Group (CLADAG 2019), Cassino, Italy, 11-19 September 2019
ISBN
978-3-030-69943-7
This item is made available under a Creative Commons License
File(s)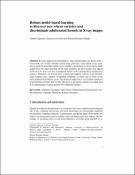
Loading...
Name
Robust model-based learning to discover new wheat varieties and discriminate adulterated kernels in X-ray images.pdf
Size
225.72 KB
Format
Adobe PDF
Checksum (MD5)
8672276a5e707eefe6d89f207e5353b0
Owning collection