Options
Calibration of conditional composite likelihood for Bayesian inference on Gibbs random fields
Author(s)
Date Issued
2015-05-12
Date Available
2015-04-29T11:50:13Z
Abstract
Gibbs random fields play an important role in statistics, however, the resulting likelihood is typically unavailable due to an intractable normalizing constant. Composite likelihoods offer a principled means to construct useful approximations. This paper provides a mean to calibrate the posterior distribution resulting from using a composite likelihood and illustrate its performance in several examples.
Sponsorship
Science Foundation Ireland
Type of Material
Journal Article
Publisher
Microtome Publishing
Journal
JMLR Workshop and Conference Proceedings
Volume
38
Start Page
921
End Page
929
Copyright (Published Version)
2015 the authors
Subjects
Language
English
Status of Item
Peer reviewed
Journal
18th International Conference on Artificial Intelligence and Statistics (AISTATS), San Diego, California, USA, 9-12 May 2015
This item is made available under a Creative Commons License
File(s)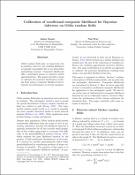
Loading...
Name
insight_publication.pdf
Size
436.76 KB
Format
Adobe PDF
Checksum (MD5)
b824c4e0dc9a62d415f18451c67735bb
Owning collection
Mapped collections