Options
Improving the accuracy of automated facial age estimation to aid CSEM investigations
Date Issued
2019-04
Date Available
2023-11-29T10:30:54Z
Abstract
The investigation of violent crimes against individuals, such as the investigation of child sexual exploitation material (CSEM), is one of the more commonly encountered criminal investigation types throughout the world. While hash lists of known CSEM content are commonly used to identify previously encountered material on suspects’ devices, previously unencountered material requires expert, manual analysis and categorisation. The discovery, analysis, and categorisation of these digital images and videos has the potential to be significantly expedited with the use of automated artificial intelligence (AI) based techniques. Intelligent, automated evidence processing and prioritisation has the potential to aid investigators in alleviating some of the digital evidence backlogs that have become commonplace worldwide. In order for AI-aided CSEM investigations to be beneficial, the fundamental question when analysing multimedia content becomes “how old is each subject encountered?’’. Our work presents the evaluation of existing cloud-based and offline age estimation services, introduces our deep learning model, DS13K, which was created with a VGG-16 Deep Convolutional Neural Network (CNN) architecture, and develops an ensemble technique that improves the accuracy of underage facial age estimation. In addition to our model, a number of existing services including Amazon Rekognition, Microsoft Azure Cognitive Services, How-Old.net, and Deep Expectation (DEX) were used to create an ensemble learning technique. It was found that for the borderline adulthood age range (i.e., 16–17 years old), our DS13K model substantially outperformed existing services, achieving a performance accuracy of 68%. A comparative examination of the obtained results allowed us to identify performance trends and issues inherent to each service/tool and develop ensemble techniques to improve the accuracy of automated adulthood determination.
Type of Material
Journal Article
Publisher
Elsevier
Journal
Digital Investigation
Volume
28
Issue
Supplement
Copyright (Published Version)
2019 the Authors
Web versions
Language
English
Status of Item
Peer reviewed
ISSN
1742-2876
This item is made available under a Creative Commons License
File(s)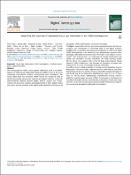
Loading...
Name
1-s2.0-S1742287619300349-main.pdf
Size
150.71 KB
Format
Adobe PDF
Checksum (MD5)
c0912d27abbf41f39988cd148ff06021
Owning collection