Options
Variable selection methods for model-based clustering
Author(s)
Date Issued
2018-04-26
Date Available
2019-05-20T09:23:26Z
Abstract
Model-based clustering is a popular approach for clustering multivariate data which has seen applications in numerous fields. Nowadays, high-dimensional data are more and more common and the model-based clustering approach has adapted to deal with the increasing dimensionality. In particular, the development of variable selection techniques has received a lot of attention and research effort in recent years. Even for small size problems, variable selection has been advocated to facilitate the interpretation of the clustering results. This review provides a summary of the methods developed for variable selection in model-based clustering. Existing R packages implementing the different methods are indicated and illustrated in application to two data analysis examples.
Sponsorship
Science Foundation Ireland
Other Sponsorship
Insight Research Centre
Type of Material
Journal Article
Publisher
The American Statistical Association, the Bernoulli Society, the Institute of Mathematical Statistics, and the Statistical Society of Canada
Journal
Statistics Surveys
Volume
12
Start Page
18
End Page
65
Copyright (Published Version)
2018 the Authors
Language
English
Status of Item
Peer reviewed
ISSN
1935-7516
This item is made available under a Creative Commons License
File(s)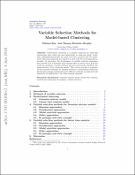
Loading...
Name
insight_publication.pdf
Size
1.23 MB
Format
Adobe PDF
Checksum (MD5)
8cd7db9750b5537780e4108bc660a6bd
Owning collection
Mapped collections