Options
Automatic detection and characterization of seizures and sleep spindles in electroencephalograms using machine learning
Author(s)
Date Issued
2022
Date Available
2022-12-07T17:22:09Z
Abstract
Electroencephalography (EEG) is an electrophysiological monitoring method used to measure tiny electrical changes of the brain, and it is commonly used in research involving neural engineering, neuroscience, and biomedical engineering. EEG is widely used to assist clinicians and researchers in analysing brain events, such as emotion recognition, sleep events identification, seizure detection, and Alzheimer’s classification. In this thesis, I describe the methods that I have developed for the detection of seizures and sleep spindle events in EEG recordings. Sixty-five million people worldwide suffer from epilepsy, and epilepsy-related disability, death, comorbidities, stigma and costs are the major burdens of epilepsy. Epilepsy is characterised by unpredictable seizures and can cause other health problems. In order to study disease development, understand disease mechanisms and evaluate the effects of anticonvulsant drugs and experimental treatments, EEG monitoring is also commonly used in rodent disease models of epilepsy. Increasingly, the field is moving toward identifying disease-modifying actions of drugs necessitating long-term recordings of EEG in rodents such as mice. Sleep spindles are significant transient oscillations in sleep stage N2; their developmental changes may be related to the maturation of thalamic cortical structures and are of considerable significance to the study of brain development in infants. However, manually identifying these brain events in EEG recordings is very time-consuming and typically requires highly trained experts. Automatic brain events detection would greatly facilitate this analysis. Research to date on automatic brain events detection methods in EEG data has been limited. The methods that I have developed have the potential to be beneficial in both experimental and clinical settings, greatly improving the speed, reliability and reproducibility of seizure and sleep spindles analysis in EEG data. Moreover, these methods were implemented as webservers that are made available for free academic use. This will assist researchers and clinicians in the automated analysis of seizures and sleep spindle events in EEG recordings.
Type of Material
Doctoral Thesis
Publisher
University College Dublin. School of Computer Science
Qualification Name
Ph.D.
Copyright (Published Version)
2022 the Author
Language
English
Status of Item
Peer reviewed
This item is made available under a Creative Commons License
File(s)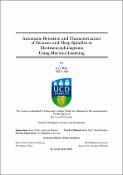
Loading...
Name
103183901.pdf
Size
7.38 MB
Format
Adobe PDF
Checksum (MD5)
f7f88880326aed11d666cafe59a7ea01
Owning collection