Options
Bayesian Personalized Ranking for Novelty Enhancement
Author(s)
Date Issued
2019-06-12
Date Available
2019-07-02T07:06:22Z
Abstract
Novelty enhancement of recommendations is typically achieved through a post-filtering process applied on a candidate set of items. While it is an effective method, its performance heavily depends on the quality of a baseline algorithm, and many of the state-of-the-art algorithms generate recommendations that are relatively similar to what the user has interacted with in the past. In this paper we explore the use of sampling as a means of novelty enhancement in the Bayesian Personalized Ranking objective. We evaluate the proposed extensions on the MovieLens 20M dataset, and show that the proposed method can be successfully used instead of two-step re-ranking, as it offers comparable and better accuracy/novelty tradeoffs, and more unique recommendations.
Sponsorship
Science Foundation Ireland
Other Sponsorship
Insight Research Centre
Type of Material
Conference Publication
Publisher
ACM
Start Page
144
End Page
148
Copyright (Published Version)
2019 Association for Computing Machinery
Web versions
Language
English
Status of Item
Peer reviewed
Journal
UMAP '19 Proceedings of the 27th ACM Conference on User Modeling, Adaptation and Personalization
Conference Details
UMAP '19: 27th ACM Conference on User Modeling, Adaptation and Personalization, Larnaca, Cyprus, 9–12 June 2019
ISBN
978-1-4503-6021-0
This item is made available under a Creative Commons License
File(s)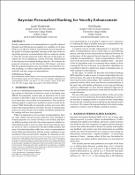
Loading...
Name
insight_publication.pdf
Size
1.73 MB
Format
Adobe PDF
Checksum (MD5)
b1e18d11f42b47e035e4ce448731838d
Owning collection