Options
Identifying online credit card fraud using artificial immune systems
Date Issued
2010-07
Date Available
2011-01-20T16:46:33Z
Abstract
Significant payment flows now take place on-line, giving rise to a requirement for efficient and effective systems for the detection of credit card fraud. A particular aspect of this problem is that it is highly dynamic, as fraudsters continually adapt their strategies in response to the increasing sophistication of detection systems. Hence, system training by exposure to examples of previous examples of fraudulent transactions can lead to fraud detection systems which are susceptible to new patterns of fraudulent transactions. The nature of the problem suggests that Artificial Immune Systems (AIS) may have particular utility for inclusion in fraud detection systems as AIS can be constructed which can flag ‘non standard’ transactions without having seen examples of all possible such transactions during training of the algorithm. In this paper, we investigate the effectiveness of Artificial Immune Systems (AIS) for credit card fraud detection using a large dataset obtained from an on-line retailer. Three AIS algorithms were implemented and their performance was benchmarked against a logistic regression model. The results suggest that AIS algorithms have potential for inclusion in fraud detection systems but that further work is required to realize their full potential in this domain.
Sponsorship
Science Foundation Ireland
Type of Material
Conference Publication
Publisher
IEEE Press
Copyright (Published Version)
2010 IEEE
Subject – LCSH
Credit card fraud
Immunocomputers
Web versions
Language
English
Status of Item
Peer reviewed
Journal
2010 IEEE Congress on Evolutionary Computation (CEC) [proceedings]
Conference Details
Congress on Evolutionary Computation, IEEE World Congress on Computational Intelligence, Barcelona, Spain, 18-23 July
ISBN
978-1-4244-6909-3
This item is made available under a Creative Commons License
File(s)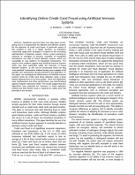
Loading...
Name
CEC_2010_AISOnlineCreditCardFraud.pdf
Size
187.2 KB
Format
Adobe PDF
Checksum (MD5)
2824eb1a44e05ae839e0f77c216d3e7e
Owning collection