Options
Automated Bridge Deck Evaluation through UAV Derived Point Cloud
Date Issued
2018-08-30
Date Available
2019-05-20T12:25:21Z
Abstract
Imagery-based, three-dimensional (3D) reconstructions from Unmanned Aerial Vehicles (UAVs) hold the potential to provide a safer, more economical, and less disruptive approach for bridge inspection. This paper describes a methodology using a low-cost UAV to generate an imagery-based, dense point cloud for bridge deck inspection. Structure from motion (SfM) is employed to create a three-dimensional (3D) point cloud. Outlier data are removed through a density-based filtering method. Next, the unsupervised learning algorithm k-means and an object-based region growing algorithm are compared for accuracy with respect to bridge deck extraction. Last, an automatic pavement evaluation method is proposed to estimate the deck’s pavement condition. The procedure is demonstrated through an actual case study, in which a 3D point cloud of 16 million valid points was generated from 212 images. With that data set, the region growing method successfully extracted the deck area with an F-score close to 95%, while the unsupervised learning approach only achieved 76%. In the last, to evaluate the surface condition of the extracted pavement, a polynomial surface fitting method was designed to evaluate and visualise the damages.
Sponsorship
European Commission
European Commission Horizon 2020
University College Dublin
Type of Material
Conference Publication
Publisher
CERAI
Copyright (Published Version)
2018 the Authors
Web versions
Language
English
Status of Item
Peer reviewed
Journal
Pakrashi, V., Keenahan, J. (eds.). Civil Engineering Research in Ireland 2018: Conference Proceedings
Conference Details
Civil Engineering Research in Ireland 2018 Conference (CERI 2018), Dublin, Ireland, 29-30 August 2018
ISBN
978-0-9573957-3-2
This item is made available under a Creative Commons License
File(s)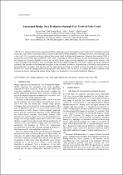
Loading...
Name
CERI_2018.pdf
Size
2.93 MB
Format
Adobe PDF
Checksum (MD5)
4cab03f146ba491c23325aca5843bdc9
Owning collection