Options
Improving the generalisation ability of genetic programming with semantic similarity based crossover
Date Issued
2010
Date Available
2010-11-22T14:38:12Z
Abstract
This paper examines the impact of semantic control on the ability of Genetic Programming (GP) to generalise via a semantic based crossover operator (Semantic Similarity based Crossover - SSC). The use of validation sets is also investigated for both standard crossover and SSC. All GP systems are tested on a number of real-valued symbolic regression problems. The experimental results show that while using validation sets barely improve generalisation ability of GP, by using semantics, the performance of Genetic Programming is enhanced both on training and testing data. Further recorded statistics shows that the size of the evolved solutions by using SSC are often smaller than ones obtained from GP systems that do not use semantics. This can be seen as one of the reasons for the success of SSC in improving the generalisation ability of GP.
Sponsorship
Irish Research Council for Science, Engineering and Technology
Type of Material
Conference Publication
Publisher
Springer
Copyright (Published Version)
2010 Springer-Verlag Berlin Heidelberg
Subject – LCSH
Genetic programming (Computer science)
Semantic computing
Natural computation
Evolutionary computation
Language
English
Status of Item
Peer reviewed
Journal
Esparcia-Alcázar, A. I. et al. (eds.). Genetic Programming 13th European Conference, EuroGP 2010, Istanbul, Turkey, April 7-9, 2010. Proceedings
Conference Details
European Conference on Genetic Programming, Istanbul Turkey, 7-9 April 2010
ISBN
978-3-642-12147-0
This item is made available under a Creative Commons License
File(s)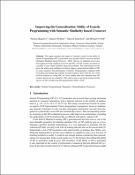
Loading...
Name
semanticsEuroGP2010.pdf
Size
80.59 KB
Format
Adobe PDF
Checksum (MD5)
887f646fe9e73122cc5d6e23b357edc0
Owning collection
Mapped collections