Options
David Versus Goliath: Fundamental Patterns and Predictions in Modern Wars and Terrorist Campaigns
Author(s)
Date Issued
2017-10
Date Available
2017-12-12T15:57:01Z
Abstract
It is still unknown whether there is some deep structure to modern wars and terrorist campaigns that could allow reliable prediction of future patterns of violent events. Recent war research focuses on size distributions of violent events, with size defined by the number of people killed in each event. Event size distributions within previously available datasets, for both armed conflicts and for global terrorism as a whole, exhibit extraordinary regularities that transcend specifics of time and place. These distributions have been well modelled by a narrow range of power laws that are, in turn, supported by a theory of coalescence and fragmentation of violent groups. We show that the predicted eventsize patterns emerge in a mass of new event data covering conflict in Africa and Asia from 1990 to 2014. Moreover, there are similar regularities in the events generated by individual terrorist organizations, 1997-2014. The existence of such robust empirical patterns hints at the predictability of size distributions of violent events in future wars. We pursue this prospect using split-sample techniques that help us to make useful out-of-sample predictions. Power-law-based prediction systems outperform lognormal-based systems. We conclude that there is indeed evidence from the existing data that fundamental patterns do exist, and that these can allow prediction of future structures in modern wars and terrorist campaigns.
Type of Material
Working Paper
Publisher
University College Dublin. School of Economics
Start Page
1
End Page
13
Series
UCD Centre for Economic Research Working Paper Series
2017/21
Copyright (Published Version)
2017 the Authors
Classification
C46
C53
D74
Language
English
Status of Item
Not peer reviewed
This item is made available under a Creative Commons License
File(s)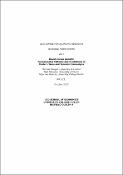
Loading...
Name
WP17_21.pdf
Size
431.96 KB
Format
Adobe PDF
Checksum (MD5)
4dc43950d14e201ed3d99c7eb6d2a1db
Owning collection