Options
Play MNIST For Me! User Studies on the Effects of Post-Hoc, Example-Based Explanations & Error Rates on Debugging a Deep Learning, Black-Box Classifier
Author(s)
Date Issued
2021-01-08
Date Available
2024-05-08T10:10:31Z
Abstract
This paper reports two experiments (N=349) on the impact of post-hoc explanations-by-example and error-rates on people’s perceptions of a black-box classifier. Both experiments show that when people are given case-based explanations, from an implemented ANN-CBR twin system, they perceive miss-classifications to be more correct. They also show that as error rates increase above ~4%, people trust the classifier less and view it as being less correct, less reasonable and less trustworthy. The
implications of these results for XAI are discussed.
implications of these results for XAI are discussed.
Sponsorship
Department of Agriculture, Food and the Marine
Science Foundation Ireland
Other Sponsorship
Insight Research Centre
Type of Material
Conference Publication
Web versions
Language
English
Status of Item
Peer reviewed
Conference Details
The IJCAI-20 Workshop on Explainable AI (XAI), Online Conference, 8 January 2021
This item is made available under a Creative Commons License
File(s)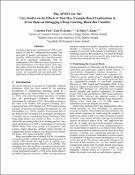
Loading...
Name
Play MNIST For Me! User Studies on the Effects of Post-Hoc, Example-Based Explanations & Error Rates on Debugging a Deep Learning, Black-Box Classifier.pdf
Size
859.07 KB
Format
Adobe PDF
Checksum (MD5)
47c3938d3a0d6b5f92d78077a753db2c
Owning collection
Mapped collections