Options
Classification of forestry species using singular value decomposition
Date Issued
1994-09
Date Available
2013-01-14T16:59:22Z
Abstract
A method is defined and tested for the classification of forest species from multi-spectral data, based on singular value decomposition (SVD) and key vector analysis. The SVD technique, which bears a close resemblance to multivariate statistic techniques has previously been successfully applied to the problem of signal extraction from marine data. In this study the SVD technique is used as a classifier for forest regions, using SPOT and landsat thematic mapper data. The specific region chosen is in the County Wicklow area of Ireland. This area has a large number of species, within a very small region and hence is not amenable to existing techniques. Preliminary results indicate that SVD is a fast and efficient classifier with the ability to differentiate between species such as Scots pine, Japanese larch and Sitka spruce. Classification accuracy's using this technique yielded excellent results of > 99% for forest, against four background classes. The accuracy's of the individual species classification are slightly lower, but they are still high at 97 - 100% for the SPOT wavebands. When the Landsat TM bands 3, 4, and 5 were used on their own, accuracies of 95 - 100% were achieved.
Type of Material
Journal Article
Publisher
The International Society for Optical Engineering
Copyright (Published Version)
1995 SPIE--The International Society for Optical Engineering
Subjects
Language
English
Status of Item
Not peer reviewed
Journal
Eric Mougin; K. Jon Ranson; James A. Smith (eds.). Multispectral and Microwave Sensing of Forestry, Hydrology, and Natural Resources (SPIE proceedings, vol. 2314)
Conference Details
Multispectral and Microwave Sensing of Forestry, Hydrology, and Natural Resources, Rome, Italy, September 26, 1994
This item is made available under a Creative Commons License
File(s)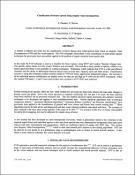
Loading...
Name
Danaher_et_al_1995.pdf
Size
265.71 KB
Format
Adobe PDF
Checksum (MD5)
2aacd52f26d9aef25fb66261bcf2a196
Owning collection