Options
The use of Bayesian Statistics to predict patterns of spatial repeatability
Date Issued
2006-10
Date Available
2010-08-10T15:17:41Z
Abstract
Statistical spatial repeatability (SSR) is an extension to the well known concept of spatial repeatability. SSR states that the mean of many patterns of dynamic tyre force applied to a pavement surface is similar for a fleet of trucks of a given type. A model which can accurately predict patterns of SSR could subsequently be used in whole-life pavement deterioration models as a means of describing pavement loading due to a fleet of vehicles. This paper presents a method for predicting patterns of SSR, through the use of a truck fleet model inferred from measurements of dynamic tyre forces. A Bayesian statistical inference algorithm is used to determine the distributions of multiple parameters of a fleet of quarter-car heavy vehicle ride models, based on prior assumed distributions and the set of observed dynamic tyre force from a ‘true’ fleet of 100 simulated models. Simulated forces are noted at 16 equidistant pavement locations, similar to data from a multiple sensor weigh-in-motion site. It is shown that the fitted model provides excellent agreement in the mean pattern of dynamic force with the originally generated truck fleet. It is shown that good predictions are possible for patterns of SSR on a given section of road for a fleet of similar vehicles. The sensitivity of the model to errors in parameter estimation is discussed, as is the potential for implementation of the method.
Sponsorship
Irish Research Council for Science, Engineering and Technology
Type of Material
Journal Article
Publisher
Elsevier
Journal
Transportation Research Part C : Emerging Technologies
Volume
14
Issue
5
Start Page
303
End Page
315
Copyright (Published Version)
2006 Elsevier Ltd
Subject – LCSH
Spatial analysis (Statistics)
Bayesian statistical decision theory
Live loads--Statistical methods
Web versions
Language
English
Status of Item
Peer reviewed
ISSN
0968-090X
This item is made available under a Creative Commons License
File(s)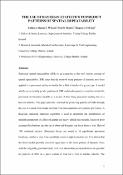
Loading...
Name
J32 Bayes-Tr-Pop_as_snt.pdf
Size
487.13 KB
Format
Adobe PDF
Checksum (MD5)
0a8af3fbe2d293291636b7cbe8031312
Owning collection
Mapped collections