Options
Improved errors-in-variables estimators for grouped data
Author(s)
Date Issued
2006-01
Date Available
2008-12-12T15:13:08Z
Abstract
Grouping models are widely used in economics but are subject to finite sample bias. I show that the standard errors-in-variables estimator (EVE) is exactly equivalent to the Jackknife Instrumental Variables Estimator (JIVE), and use this relationship to develop an estimator which, unlike EVE, is unbiased in finite samples.
The theoretical results are demonstrated using Monte Carlo experiments. Finally, I implement a model of intertemporal male labor supply using microdata from the United States Census. There are sizeable differences in the wage elasticity across estimators, showing the practical importance of the theoretical issues even when the sample size is quite large.
The theoretical results are demonstrated using Monte Carlo experiments. Finally, I implement a model of intertemporal male labor supply using microdata from the United States Census. There are sizeable differences in the wage elasticity across estimators, showing the practical importance of the theoretical issues even when the sample size is quite large.
Type of Material
Working Paper
Publisher
University College Dublin. School of Economics
Series
UCD Centre for Economic Research Working Paper Series
WP06/02
Copyright (Published Version)
UCD School of Economics 2006
Subject – LCSH
Labor supply--Mathematical models
Jackknife (Statistics)
Monte Carlo method
Language
English
Status of Item
Not peer reviewed
This item is made available under a Creative Commons License
File(s)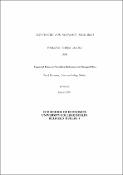
Loading...
Name
devereuxp_workpap_010.pdf
Size
259.93 KB
Format
Adobe PDF
Checksum (MD5)
923f73e7dbb0f6623baa1fafa17d4424
Owning collection