Options
Predicting helpful product reviews
Date Issued
2010-08-30
Date Available
2010-10-12T15:14:02Z
Abstract
Millions of users are today posting user-generated content online, expressing their opinions on all manner of goods and services, topics and social affairs. While undoubtedly useful,user-generated content presents consumers with significant challenges in terms of information overload and quality considerations. In this paper, we address
these issues in the context of product reviews and present a brief survey of our work to date on predicting review helpfulness. In particular, the performance of a variety of different machine learning approaches is evaluated on four large-scale review datasets drawn from the TripAdvisor
and Amazon domains. Our findings highlight some interesting properties of this task from a machine learning perspective and demonstrate that author reputation, the sentiment expressed in reviews and review length are among the most effective predictors of review helpfulness.
these issues in the context of product reviews and present a brief survey of our work to date on predicting review helpfulness. In particular, the performance of a variety of different machine learning approaches is evaluated on four large-scale review datasets drawn from the TripAdvisor
and Amazon domains. Our findings highlight some interesting properties of this task from a machine learning perspective and demonstrate that author reputation, the sentiment expressed in reviews and review length are among the most effective predictors of review helpfulness.
Sponsorship
Science Foundation Ireland
Type of Material
Conference Publication
Subject – LCSH
User-generated content--Evaluation
Recommender systems (Information filtering)
User-generated content--Classification
Language
English
Status of Item
Peer reviewed
Conference Details
Paper presented at the 21st Irish Conference on Artificial Intelligence and Cognitive Science (AICS 2010), Galway, Ireland, 30 August - 1 September, 2010
This item is made available under a Creative Commons License
File(s)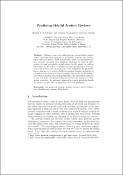
Loading...
Name
aics-2010b-crc.pdf
Size
337.59 KB
Format
Adobe PDF
Checksum (MD5)
f3e6aa8a7cffc61087e363b007b172c9
Owning collection
Mapped collections